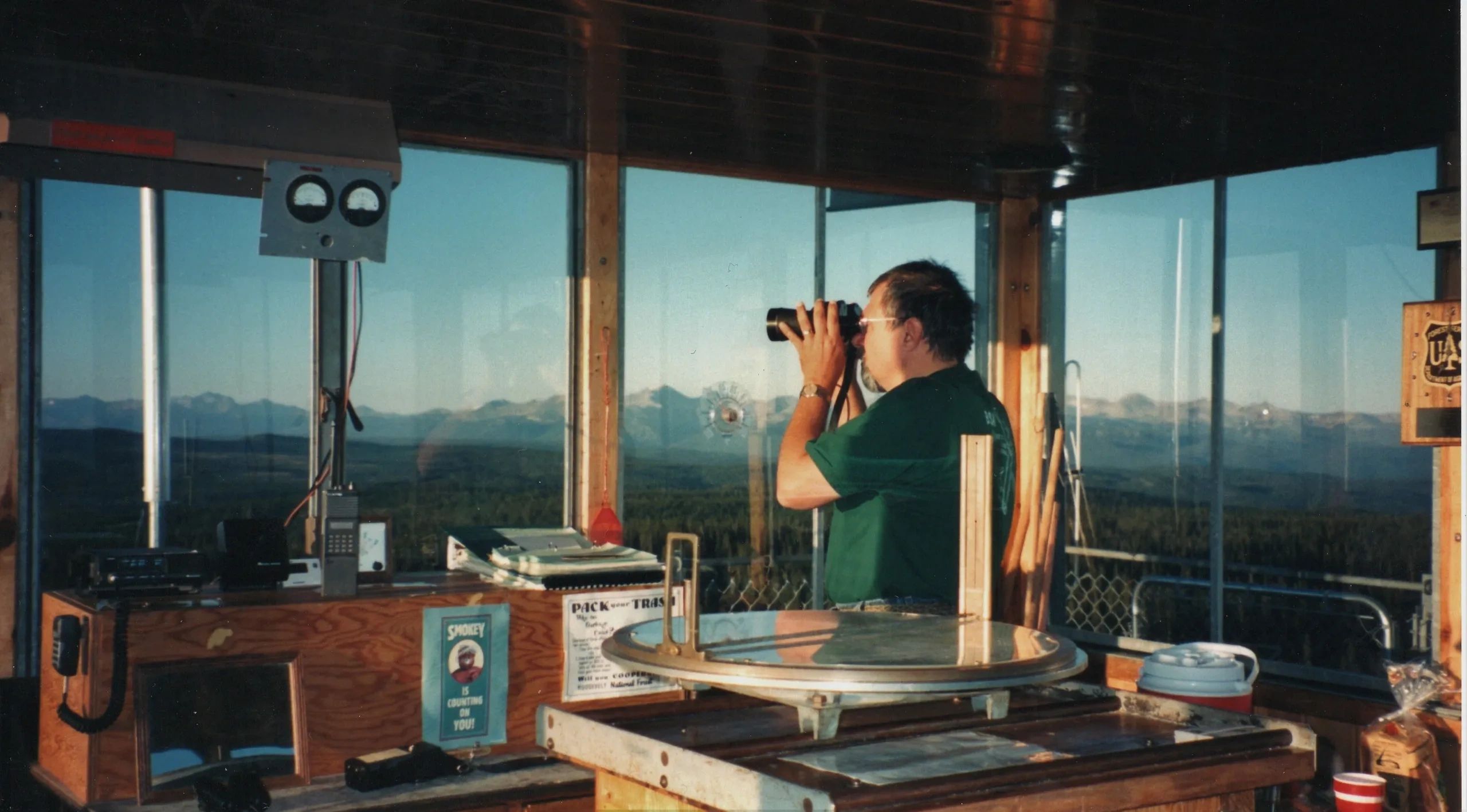
The Generalized Multivariate Difference estimator (GMDe) is a pioneering alternative to the conventional mixed estimator, calibration estimator, and regression estimator, e.g., GREG. GMDe is a recent generalization of the long neglected class of difference estimators. It is a type of data assimilation method that is closely related to the Kalman filter update.
GMDe a simple, robust, model-based method in complex sample surveys with high dimensions. GMDe improves population estimates for study variables, which serve the analysis objectives, using population estimates for auxiliary variables that are well correlated with the study variables. It is a type of composite estimator that weights population estimates rather than sampling units.
Environmetrika develops multivariate time-series estimators for complex sample surveys that monitor large environmental and ecological systems. These statistical methods are relevant to complex sample surveys for extensive biological, physical, and social systems; and accuracy assessments of remotely sensed data. Statistical methods are closely related to the Kalman filter, which supports applications such as navigation and control systems, robotics and artificial intelligence.
Environmetrika primarily supports sample surveys that use repeated measurements of population elements over time, where those elements are selected with probability sampling. These supply time-series of population estimates for "study variables" that serve an analyst’s objectives.
Population statistics use
Environmetrika primarily supports sample surveys that use repeated measurements of population elements over time, where those elements are selected with probability sampling. These supply time-series of population estimates for "study variables" that serve an analyst’s objectives.
Population statistics use
In the special case of monitoring terrestrial systems, measurements are field observations or very high resolution remotely sensed data for a probability sample of monitoring sites.
However, population estimates for study variables might not be sufficiently accurate for the analyst’s objectives.
Environmetrika develops and applies statistical estimators that improve sample survey estimates for study variables with correlated estimates for "auxiliary variables."
Measurements of auxiliary variables use relatively inexpensive technologies. Examples include low- and medium-resolution remote sensing, full-coverage predictions with geo
Environmetrika develops and applies statistical estimators that improve sample survey estimates for study variables with correlated estimates for "auxiliary variables."
Measurements of auxiliary variables use relatively inexpensive technologies. Examples include low- and medium-resolution remote sensing, full-coverage predictions with geospatial models, deterministic ecosystem models that predict expected changes over time, administrative records, independent surveys from other sources, and others. Therefore, population estimates for auxiliary variables can be more precise than those for study variables. However, auxiliary variables are imperfectly correlated with study variables, and therefore, they do not directly satisfy the the analyst's objectives.
Environmetrika develops statistical estimators that use population estimates for auxiliary variables to improve population estimates for study variables, which do directly satisfy the the analyst's objectives.
These methods replace model-assisted regression estimators with linear transformations of estimators for stochastic systems, where probability sampling is the stochastic process.
Environmetrika develops statistical methods that use a multivariate difference estimator, which is the foundation for model-assisted regression estimators in sample surveys. These methods employ mature numerical methods, such as those developed by system engineers for digital signal processing.
Environmetrika supports multivariate sample
Environmetrika develops statistical methods that use a multivariate difference estimator, which is the foundation for model-assisted regression estimators in sample surveys. These methods employ mature numerical methods, such as those developed by system engineers for digital signal processing.
Environmetrika supports multivariate sample survey estimates for thousands of study variables with thousands of auxiliary variables, including very large covariance matrices. Those estimators are robust, numerically stable and computationally efficient. A step-wise approach accommodates covariance matrices that are ill-conditioned or rank deficient.
This combination of the sample survey and system engineering disciplines produces relatively simple solutions to large and complex analyses.
We use cookies to analyze website traffic and optimize your website experience. By accepting our use of cookies, your data will be aggregated with all other user data.